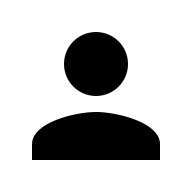
Scaling Transformer to 1M tokens and beyond with RMTの日本語訳を教えて!
こういった悩みにお答えします.
本記事の信頼性
- リアルタイムシステムの研究歴12年.
- 東大教員の時に,英語でOS(Linuxカーネル)の授業.
- 2012年9月~2013年8月にアメリカのノースカロライナ大学チャペルヒル校(UNC)コンピュータサイエンス学部で客員研究員として勤務.C言語でリアルタイムLinuxの研究開発.
- プログラミング歴15年以上,習得している言語: C/C++,Python,Solidity/Vyper,Java,Ruby,Go,Rust,D,HTML/CSS/JS/PHP,MATLAB,Verse(UEFN), Assembler (x64,aarch64).
- 東大教員の時に,C++言語で開発した「LLVMコンパイラの拡張」,C言語で開発した独自のリアルタイムOS「Mcube Kernel」をGitHubにオープンソースとして公開.
- 2020年1月~現在はアメリカのノースカロライナ州チャペルヒルにあるGuarantee Happiness LLCのCTOとしてECサイト開発やWeb/SNSマーケティングの業務.2022年6月~現在はアメリカのノースカロライナ州チャペルヒルにあるJapanese Tar Heel, Inc.のCEO兼CTO.
- 最近は自然言語処理AIとイーサリアムに関する有益な情報発信や,Unreal Editor for Fortnite(UEFN)でゲーム開発に従事.
- (AI全般を含む)自然言語処理AIの論文の日本語訳や,AIチャットボット(ChatGPT,Auto-GPT,Gemini(旧Bard)など)の記事を50本以上執筆.アメリカのサンフランシスコ(広義のシリコンバレー)の会社でChatGPT/Geminiを訓練するプロンプトエンジニア・マネージャー・Quality Assurance(QA)の業務委託の経験あり.
- (スマートコントラクトのプログラミングを含む)イーサリアムや仮想通貨全般の記事を200本以上執筆.イギリスのロンドンの会社で仮想通貨の英語の記事を日本語に翻訳する業務委託の経験あり.
- UEFNで10本以上のゲームを開発し,フォートナイト上で公開(Fortnite,Fortnite.GG).
こういった私から学べます.
AIのプログラミング言語「C++/Python言語」を学べるおすすめのWebサイトを知りたいあなたはこちらからどうぞ.
独学が難しいあなたは,AIを学べるオンラインプログラミングスクール3社で自分に合うスクールを見つけましょう.後悔はさせません!
国内・海外のAIエンジニアのおすすめ求人サイトを知りたいあなたはこちらからどうぞ. こういった悩みにお答えします. こういった私が解説していきます. 国内・海外のAIエンジニアのおすすめ求人サイト(転職エージェント)を紹介します. AIエンジニアになるためには,主にC++/Pytho ... 続きを見る
国内・海外のAIエンジニアのおすすめ求人サイト【転職エージェント】【C++/Python言語】
国内・海外のプロンプトエンジニアのおすすめ求人サイトを知りたいあなたはこちらからどうぞ.
Scaling Transformer to 1M tokens and beyond with RMTの日本語訳を紹介します.
100万トークン以上をサポートしたTransformerがわかります.
※図表を含む論文の著作権はScaling Transformer to 1M tokens and beyond with RMTの著者に帰属します.
Scaling Transformer to 1M tokens and beyond with RMTの目次は以下になります.
- Abstract
- 1章:Introduction
- 2章:Recurrent Memory Transformer
- 3章:Memorization Tasks
- 4章:Experiments
- 5章:Attention Patterns of Memory Operations
- 6章:Related work
- 7章:Discussion
- References
Scaling Transformer to 1M tokens and beyond with RMTを解説しつつ,私の考えも語ります.
Scaling Transformer to 1M tokens and beyond with RMTの概要と私の日本語訳は以下になります.
This technical report presents the application of a recurrent memory to extend the context length of BERT, one of the most effective Transformer-based models in natural language processing.
本テクニカルレポートは,自然言語処理において最も効果的なTransformerベースのモデルの一つであるBERTの文脈長を拡張するための再帰記憶のアプリケーションを提案する.By leveraging the Recurrent Memory Transformer architecture, we have successfully increased the model's effective context length to an unprecedented two million tokens, while maintaining high memory retrieval accuracy.
Recurrent Memory Transformerアーキテクチャを活用することで,高い記憶検索の正解率を維持しながら,モデルの有効文脈長を前例のない200万トークンに増やすことに成功した.Our method allows for the storage and processing of both local and global information and enables information flow between segments of the input sequence through the use of recurrence.
本手法は,ローカル情報とグローバル情報の両方を保存・処理することができ,再帰性を利用することで入力シーケンスのセグメント間の情報フローを可能にする.Our experiments demonstrate the effectiveness of our approach, which holds significant potential to enhance long-term dependency handling in natural language understanding and generation tasks as well as enable large-scale context processing for memory-intensive applications.
この手法は,自然言語理解・生成タスクにおける長期的な依存関係の処理を強化し,記憶負荷の高いアプリケーションにおける大規模なコンテキスト処理を可能にする大きな可能性を秘めていることが,実験によって証明された.https://arxiv.org/abs/2304.11062
私の日本語訳の注意点は以下になります.
- 概要は英語と日本語を両方掲載しましたが,本文は私の日本語訳のみを掲載していること(英語で読みたいあなたは原文を読みましょう!)
- 基本的には原文の直訳ですが,わかりにくい箇所は意訳や説明を追加している箇所があること
- 本文中に登場する表記「(OpenAI, 2023)」などは参考文献ですので,興味がある方は本記事の参考文献を参照されたいこと
それでは,Scaling Transformer to 1M tokens and beyond with RMTの本文を読みすすめましょう!
目次
1章:Introduction(はじめに)
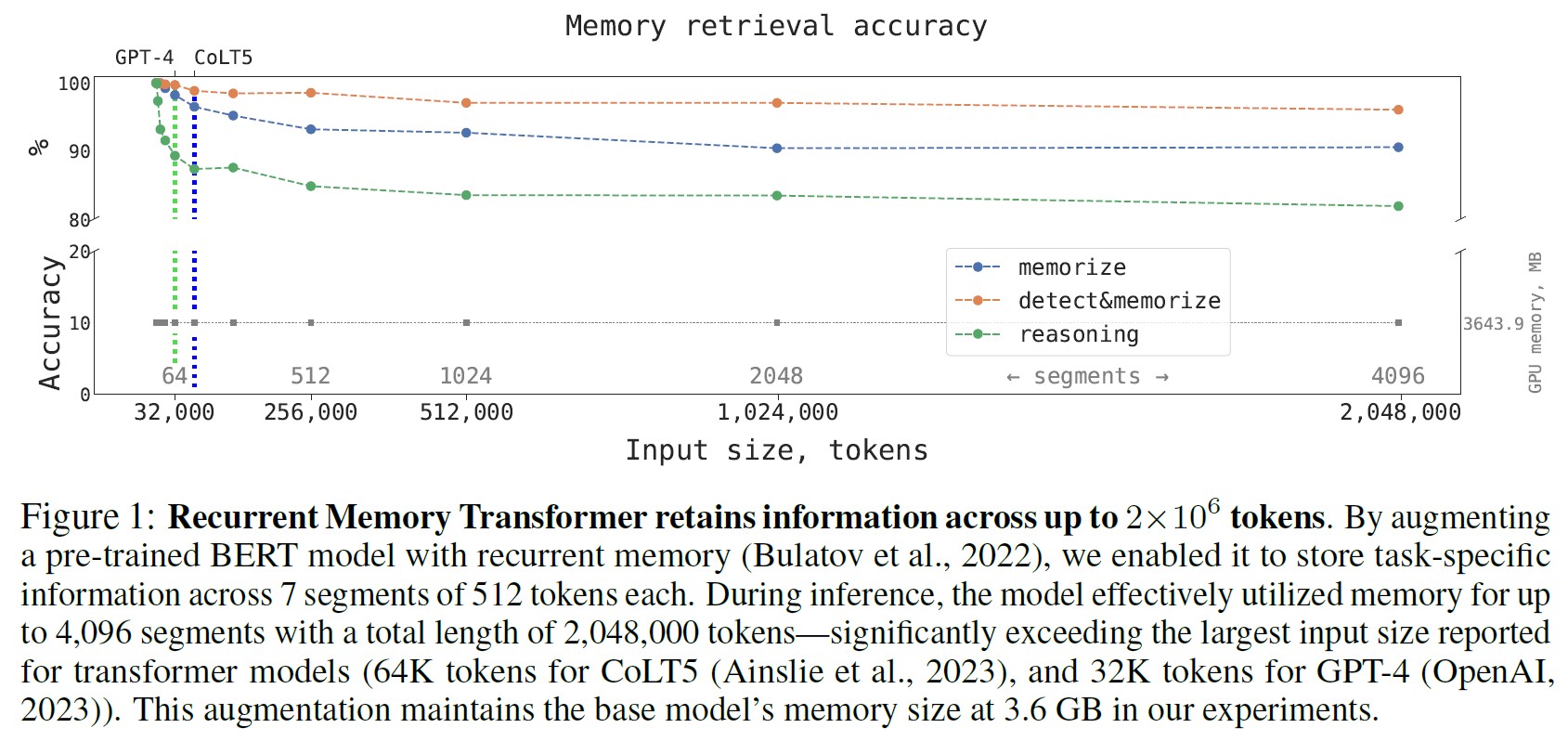
Transformerモデル(Vaswani et al., 2017)は,様々な研究分野や産業アプリケーションで広く採用され,使用されている.
このモデルの最も重要な問題は,Attention操作の2次関数的な複雑さであり,大規模なモデルをより長い入力に適用することがますます難しくなっている.
本レポートでは,(Bulatov et al., 2022)で紹介されたシンプルなトークンベースの記憶機構を用いることで,BERT(Devlin et al., 2019)のような事前訓練済みの変換モデルと組み合わせ,Full Attentionと完全精度操作により,Nvidia GTX 1080Ti GPU1台を使用して100万トークンを超えるシーケンスに適用できることを示す.
本レポートの貢献:
- トークンベースの記憶ストレージとRecurrent Memory Transformer(RMT)によるセグメントレベルの再帰を取り入れることで,BERTを強化した.
- 記憶拡張BERTは,当初設計した入力長(512トークン)の7倍までの長さのシーケンスのタスクに対処できるように訓練できることを実証した.
- 訓練されたRMTは,100万トークンを超えるような様々な長さのタスクに対しても,必要な計算量を線形にスケーリングしてうまく外挿できることを発見した.
- Attentionパターン解析により,RMTが非常に長いシーケンスを処理するために必要な,記憶に関する操作を発見した.
2章:Recurrent Memory Transformer
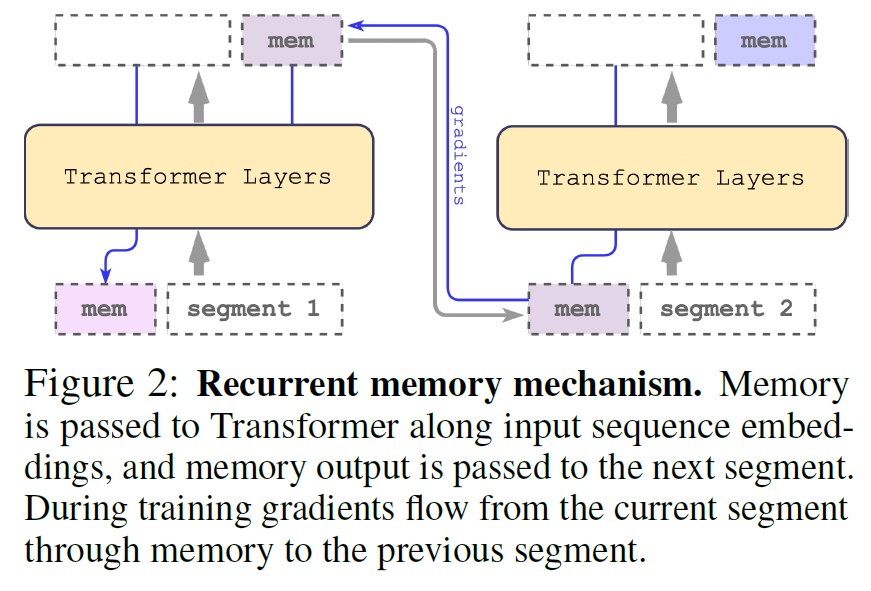
最初のRecurrent Memory Transformer(Bulatov et al., 2022)(RMT)から始めて,我々はそれをプラグアンドプレイのアプローチで,様々な人気のあるTransformerのラッパーとして適応させた.
この適応は,そのバックボーンを,m個の実数値の訓練可能なベクトルからなる記憶で拡張するものである(図2).
長い入力はセグメントに分割され,記憶ベクトルは最初のセグメント埋め込みに付加され,セグメントトークンとともに処理される.
BERTのようなエンコーダのみのモデルでは,デコーダのみのモデルが記憶を読み出し部と書き込み部に分けている(Bulatov et al., 2022)とは異なり,記憶はセグメントの最初に1回だけ追加される.
時間ステップ\(\tau\)とセグメント\(H_\tau^0\)に対して,リカレントステップは次のように実行される.
$$\tilde{H}_\tau^0 = [H_\tau^{mem} \circ H_\tau^0], \bar{H}_\tau^N = Transformer(\tilde{H}_\tau^0), [\bar{H}_\tau^{mem} \circ H_\tau^N] := \bar{H}_\tau^N$$
ここでNはTransformerの層数である.
フォワードパスの後,\(\bar{H}_\tau^{mem}\)は,セグメント\(\tau\)の更新された記憶トークンを含む.
入力シーケンスのセグメントは,順次処理される.
リカレント接続を可能にするために,現在のセグメントからの記憶トークンの出力を,次のセグメントの入力に渡す.
$$H_{\tau+1}^{mem} := \bar{H}_\tau^{mem}, \tilde{H}_{\tau+1}^0 = [H_{\tau+1}^{mem} \circ H_{\tau+1}^0]$$
RMTの記憶と再帰は,いずれもグローバル記憶トークンのみに基づいている.
これにより,バックボーンのTransformerは変更されないため,RMTの記憶拡張はTransformerファミリーのどのモデルとも互換性がある.
2.1節:Computational efficiency(計算効率)
サイズやシーケンス長の異なるRMTモデルやTransformerモデルについて,必要なFLOPsを推定することができる.
OPTモデルファミリー(Zhang et al., 2022)の構成(語彙サイズ,層数,隠れサイズ,中間隠れサイズ,Attention Heads数)をとり,(Hoffmann et al., 2022)に従いフォワードパスのFLOP数を計算した.
また,RMTの再帰の影響を考慮し,FLOPの推定値を修正した.
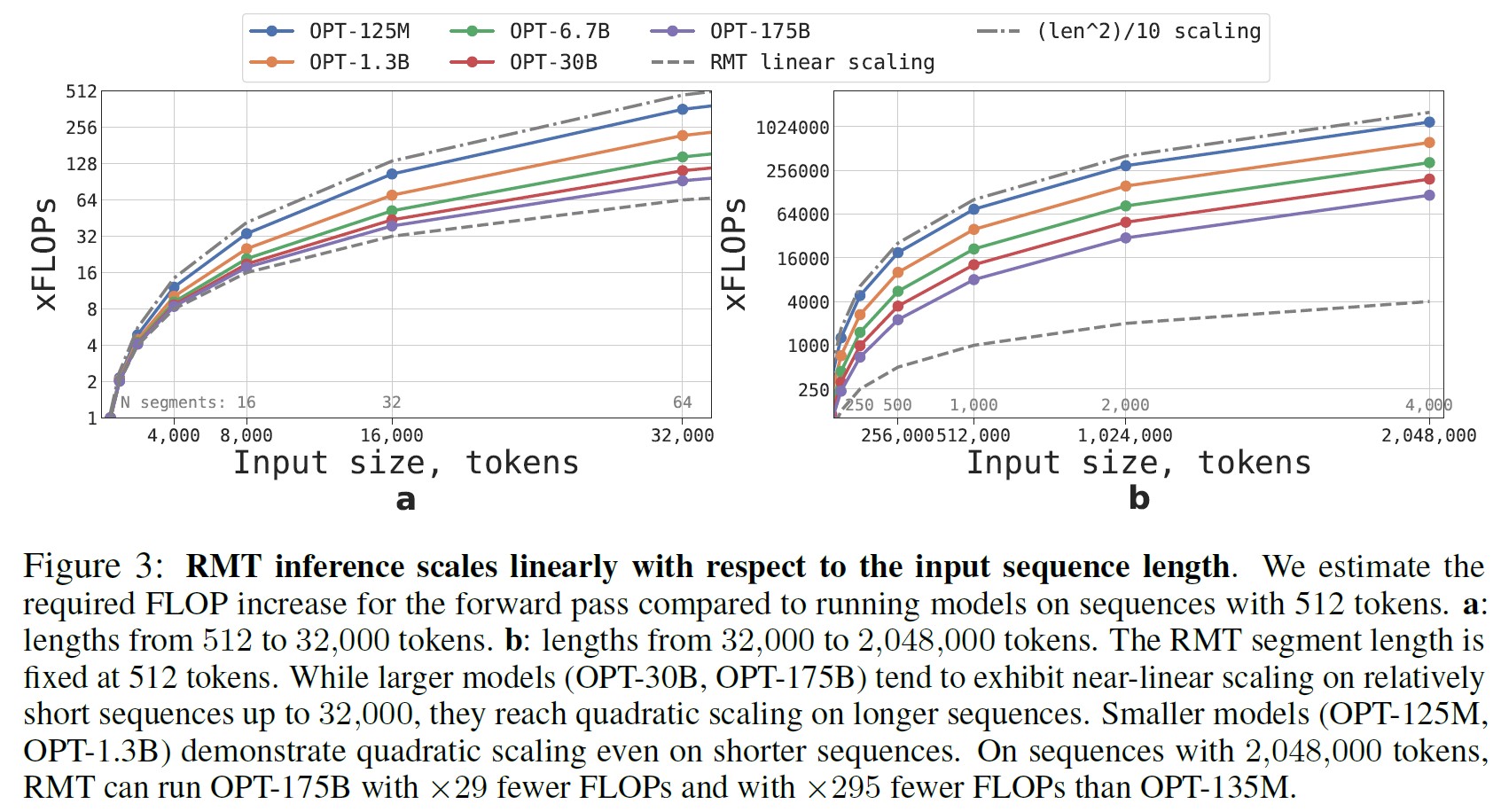
図3は,セグメント長を固定すれば,どのようなモデルサイズでもRMTが線形にスケールすることを示している.
入力シーケンスをセグメントに分割し,セグメント境界でのみFull Attention行列を計算することで,線形スケーリングを実現する.
より大きなTransformerモデルは,計算量の多いFFN層(隠れサイズに対して2次関数的にスケールする)のために,シーケンス長に対して緩やかに2次関数的なスケーリングを示す傾向がある.
しかし,32,000を超える非常に長いシーケンスでは,2次関数的なスケーリングに戻る.
RMTは,1つ以上のセグメントを持つシーケンス(本研究では512以上)において,非リカレントモデルよりも少ないFLOPs数で済み,最大で295倍までFLOsP数を減らすことができる.
RMTは,小さいモデルほどFLOPsの相対的な削減量が大きくなるが,絶対数ではOPT-175Bモデルで29倍の削減となり,非常に有意である.
3章:Memorization Tasks(記憶タスク)
記憶能力をテストするために,簡単な事実と基本的な推論の記憶を必要とする合成データセットを作成した.
タスクの入力は,1つまたは複数の事実と,これらの事実をすべて使用することによってのみ答えられる質問で構成される.
タスクの難易度を上げるために,質問や回答とは関係のない自然言語のテキストを追加した.
このテキストはノイズとして機能するため,モデルのタスクは,事実と無関係なテキストを分離し,質問に答えるためにそれらを使用することである.
タスクは6クラス分類として定式化され,各クラスは個別の回答選択肢を表す.
事実はbAbIデータセット(Weston et al., 2016)を用いて生成され,バックグラウンドテキストはQuALITY(Pang et al., 2022)ロングQAデータセットの質問から供給される.
1 2 |
Background text: ... He was a big man, broad-shouldered and still thin-waisted. Eddie found it easy to believe the stories he had heard about his father ... |
3.1節:Fact Memorization(事実記憶)
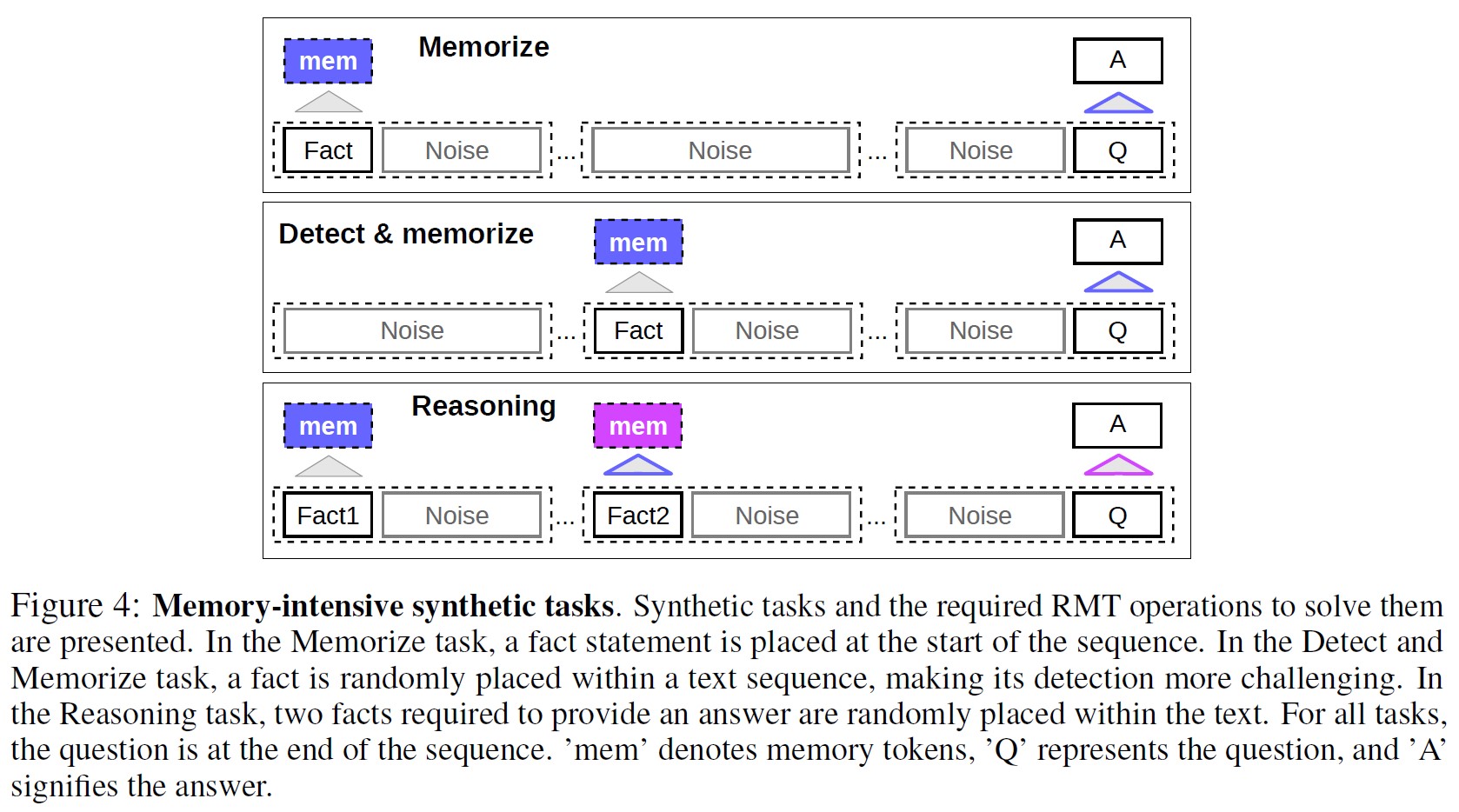
最初のタスクは,RMTが情報を書き込んで長時間記憶する能力を試すものである(図4上).
最も単純なケースでは,事実は常に入力の先頭に位置し,質問は常に末尾に位置する.
質問と回答の間にある無関係なテキストの量は徐々に増えていき,入力全体が1つのモデル入力に収まらなくなる.
1 2 3 |
Fact: Daniel went back to the hallway. Question: Where is Daniel? Answer: hallway |
3.2節:Fact Detection & Memorization(事実の検出と記憶)
事実の検出は,事実を入力のランダムな位置に移動させることでタスクの難易度を上げる(図4中).
このため,モデルはまず事実を無関係なテキストから区別し,それを記憶に書き込み,その後,末尾にある質問に答えるためにそれを使用する必要がある.
3.3節:Reasoning with Memorized Facts(記憶した事実を用いた論法)
記憶に関するもう一つの重要な操作は,記憶した事実と現在の文脈を用いた論法である.
この機能を評価するために,より複雑なタスクを使用する.
2つの事実が生成され,入力シーケンス内にランダムに配置される(図4下).
入力シーケンスの最後に提示される質問は,質問に正しく答えるためには,どの事実も使用しなければならないように定式化されている(すなわち,Two Argument Relation bAbIタスクである).
1 2 3 4 |
Fact1: The hallway is east of the bathroom. Fact2: The bedroom is west of the bathroom. Question: What is the bathroom east of? Answer: bedroom |
4章:Experiments(実験)
すべての実験でRMTのバックボーンとして,HuggingFace Transformers(Wolf et al., 2020)の事前訓練済みbert-base-casedモデルを使用する.
すべてのモデルは,記憶サイズ10で拡張され,線形学習率スケジューリングとウォームアップでAdamWオプティマイザ(Loshchilov and Hutter, 2019)を使用して訓練される.
完全な訓練パラメータは,GitHubリポジトリの訓練スクリプトで利用できるようになる予定である.
4~8台のNvidia 1080ti GPUを使用してモデルの訓練と評価を行っている.
長いシーケンスの場合は,40GBのNvidia A100に切り替えることで評価を高速化する.
4.1節:Curriculum Learning(カリキュラム学習)
訓練スケジュールを用いることで,解の正解率と安定性が大幅に向上することが確認された.
最初は,RMTはタスクの短いバージョンで訓練され,訓練が収束すると,1つのセグメントを追加することによってタスク長を増加させる.
カリキュラム学習プロセスは,希望する入力長に達するまで続けられる.
実験では,まず,1つのセグメントに収まるシーケンスから始める.
BERTの3つの特別なトークンと記憶用の10個のプレースホルダが512サイズのモデル入力から予約されているため,実用的なセグメントサイズは499である.
短いタスクで訓練した後,RMTはより少ない訓練ステップで完全な解に収束するため,より長いバージョンを解くことが容易であることに気づく.
4.2節:Extrapolation Abilities(外挿能力)
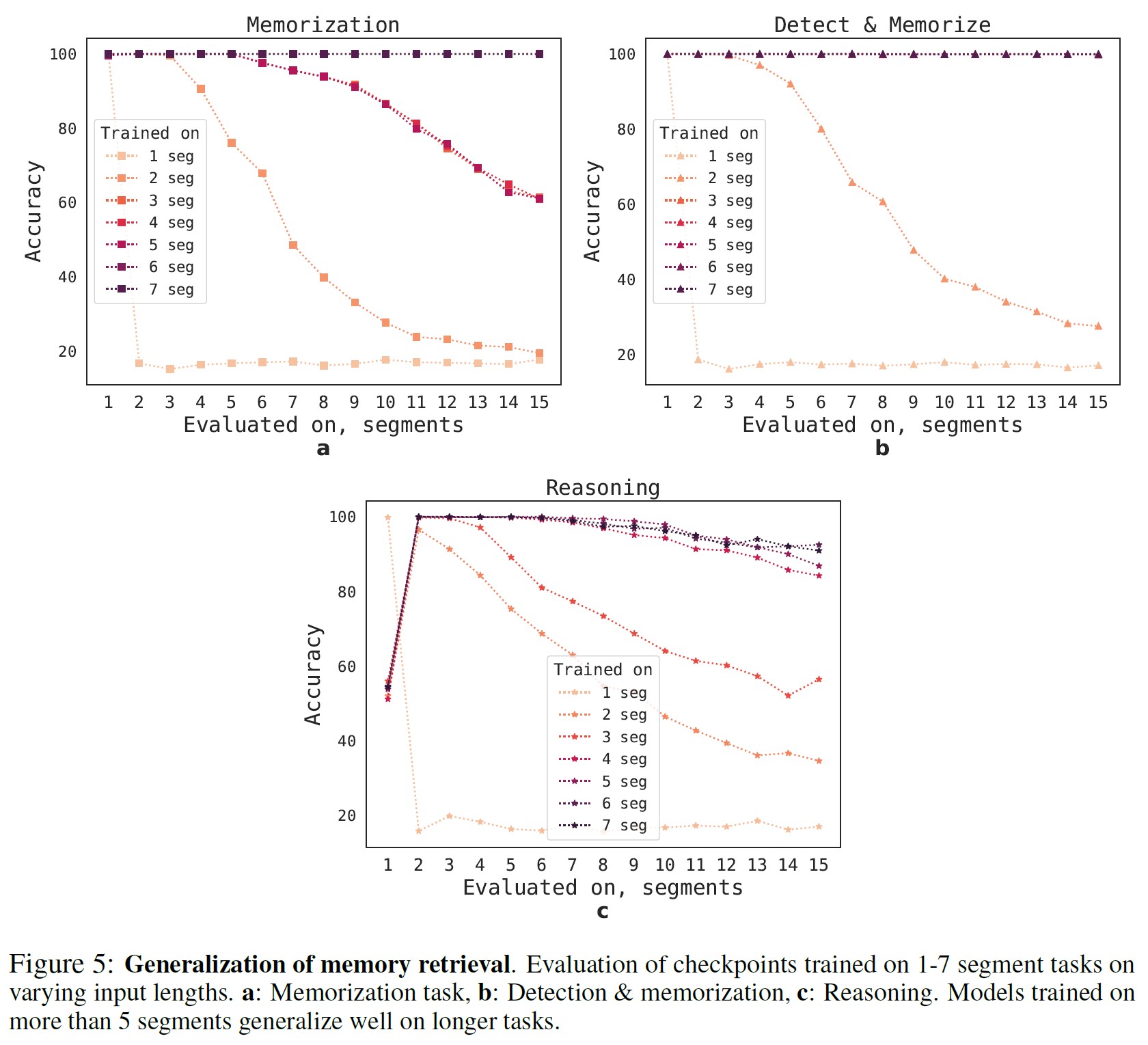
RMTは,異なる長さのシーケンス長に対してどの程度汎化できるのだろうか?
この問いに答えるため,様々な数のセグメントで訓練させたモデルを,より長い長さのタスクで評価した(図5).
その結果,短いタスクでは,モデルがうまく機能する傾向があることがわかった.
唯一の例外は,単一セグメントの推論タスクで,このタスクは,長いシーケンスでモデルを訓練させると解くことが難しくなる.
これは,タスクのサイズが1セグメントを超えるため,モデルが最初のセグメントで質問を予想しなくなり,品質が低下したためと考えられる.
興味深いことに,RMTがより長いシーケンスに汎化する能力は,訓練セグメントの数が増えるにつれても現れる.
5つより多いセグメントで訓練すると,RMTは2倍の長さのタスクに対してほぼ完璧に汎化できるようになる.
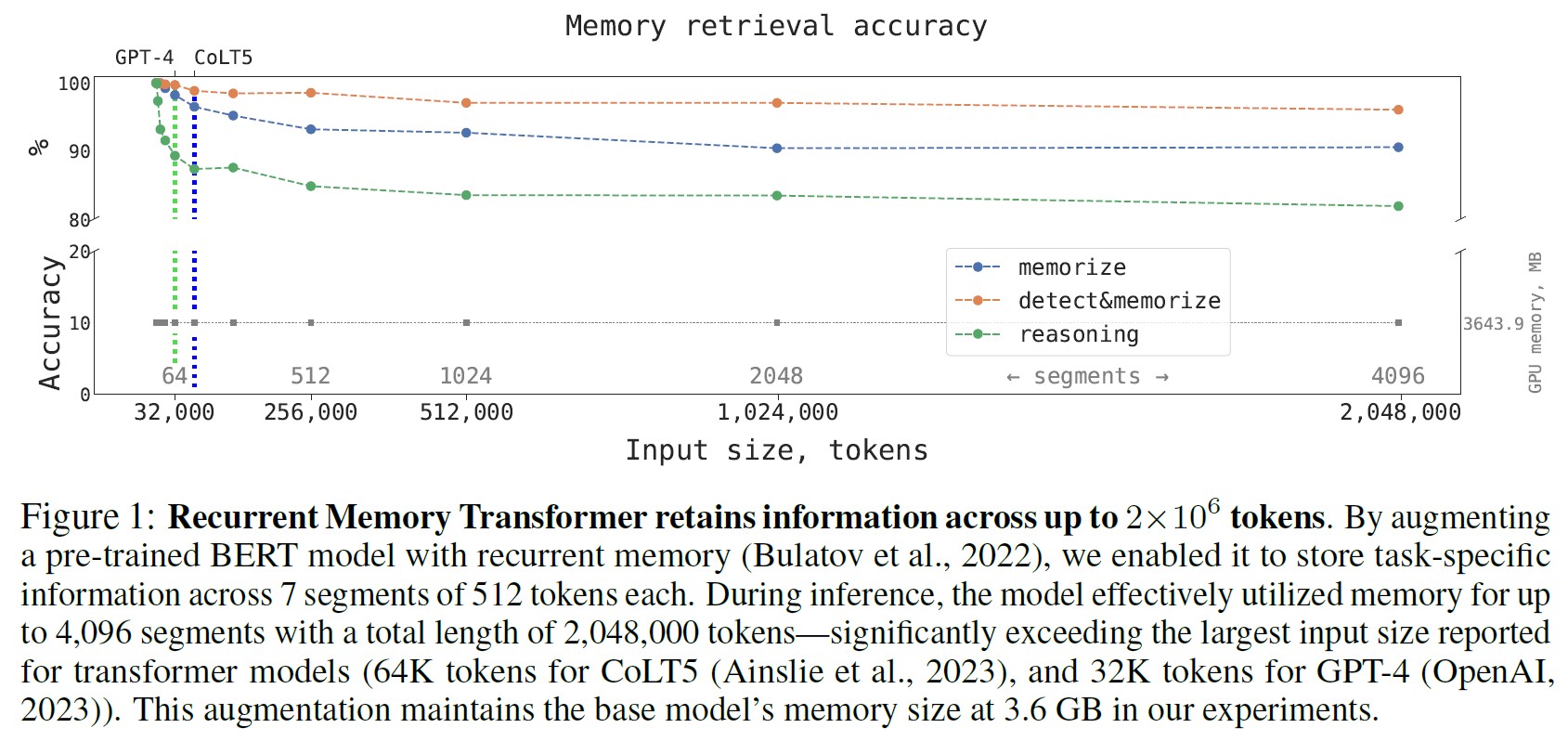
汎化の限界を検証するため,検証タスクのサイズを4096セグメント,2,043,904トークンまで拡大した(図1).
RMTは,このような長いシーケンスでも,検出・記憶タスクが最も簡単で,推論タスクが最も複雑であることから,驚くほどよく持ちこたえた.
5章:Attention Patterns of Memory Operations(記憶操作のAttentionパターン)
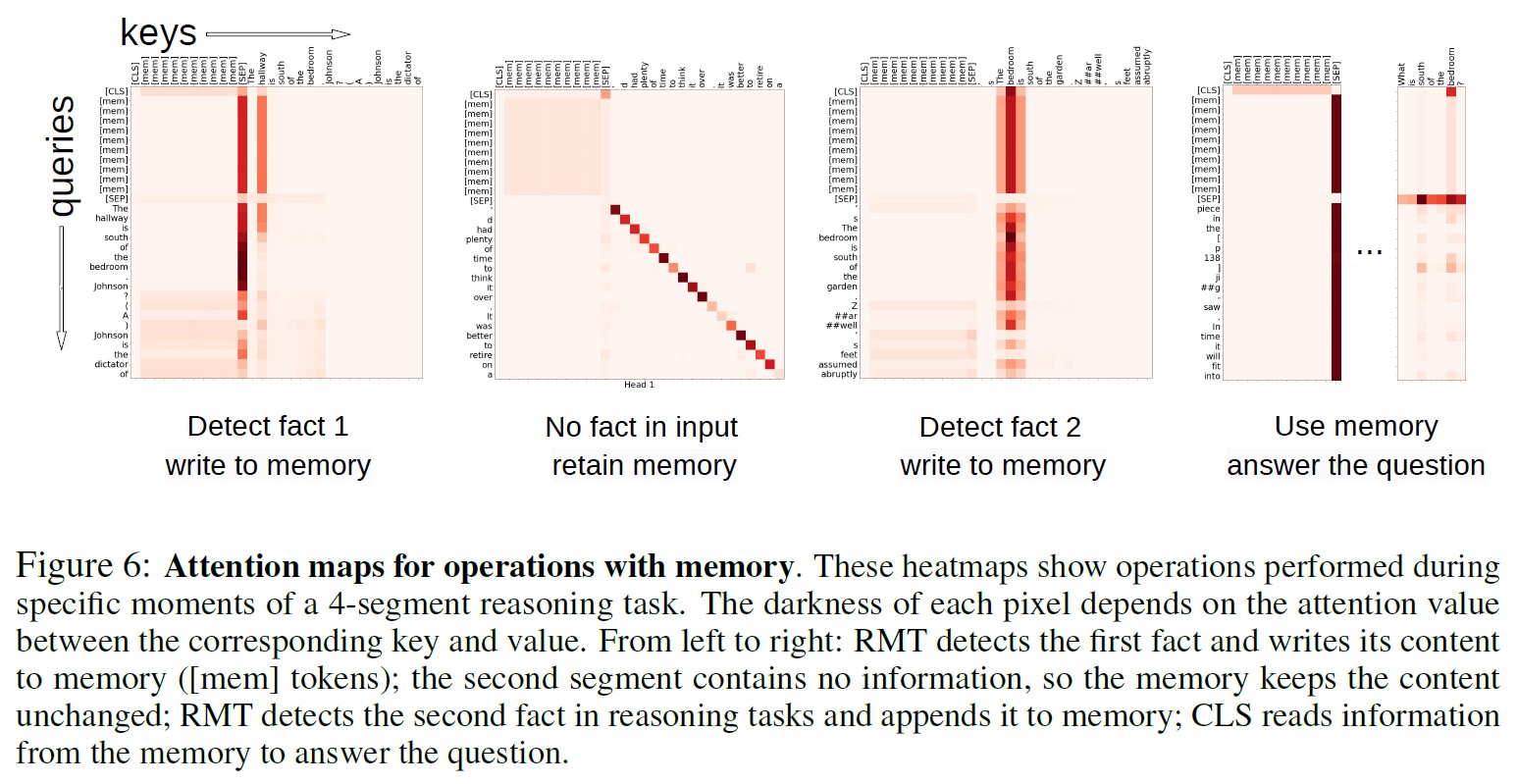
図6に示すように,特定のセグメントに対するRMTのAttentionを調べることで,記憶操作がAttentionの特定のパターンに対応していることが観察される.
さらに,4.2節で紹介したような非常に長いシーケンスに対する高い外挿性能は,数千回使用した場合でも,学習した記憶操作の有効性を実証するものである.
※訳注:原文のSection 5.2(5.2節)は4.2節の間違い.
これは,これらの操作がタスク損失によって明示的に動機づけられたものではないことを考慮すると,特に印象的である.
6章:Related work(関連研究)
我々の研究は,ニューラルアーキテクチャにおける記憶の概念を中心に展開されている.
記憶はニューラルネットワークの研究において繰り返し取り上げられるテーマであり,初期の研究(McCulloch and Pitts, 1943; Stephen, 1956)に遡り,1990年代にはBackpropagation Through Time学習アルゴリズム(Werbos, 1990)やLong-Short Term Memory(LSTM)神経アーキテクチャ(Hochreiter and Schmidhuber, 1997)を導入して大きく発展した.
現代の記憶拡張ニューラルネットワーク(MANNs:Memory-Augmented Neural Networks)は,通常,モデルのパラメータとは別に,何らかの形でリカレントな外部記憶を利用する.
ニューラルチューリングマシン(NTMs:Neural Turing Machines)(Graves et al., 2014)と記憶ネットワーク(Memory Networks)(Weston et al., 2015)は,Attention機構を通じてアクセスできるベクトル表現のストレージを備えている.
Memory Networks(Weston et al., 2015; Sukhbaatar et al., 2015)は,記憶内容に対するSequential Attentionを通じて推論を可能にするように設計された.
NTMsに続いて,Differentiable Neural Computer(DNC)(Graves et al., 2016)とSparse DNC(Rae et al., 2016)は,時間をかけて記憶ストレージに書き込むことができるリカレントニューラルネットワークとして実装されている.
これらのモデルはすべて微分可能であり,BackPropagation Through Time(BPTT)を介して訓練可能である.
並列に行われる研究ラインでは,LSTMなどのリカレントニューラルネットワークを,スタック,リスト,キューなどのデータ構造で拡張している(Joulin and Mikolov, 2015; Grefenstette et al., 2015).
アドレス-コンテンツ分離やマルチステップアドレッシングなど,より高度なアドレッシング機構を持つMANNアーキテクチャが,(Gulcehre et al., 2016, 2017; Meng and Rumshisky, 2018)において提案されている.
Global Context Layerモデル(Meng and Rumshisky, 2018)は,標準的なNTMsにおけるコンテンツベースのアドレッシングを訓練するという課題に対処するために,アドレス-コンテンツ分離を採用している.
記憶はしばしばリカレントアプローチでTransformerと組み合わされる.
長い入力は小さなセグメントに分割され,過去のセグメントからの情報にアクセスするために記憶でシーケンス処理される.
Transformer-XL(Dai et al., 2019)は,後続のセグメントで再利用するために以前の隠れ状態を保存し,Compressive Transformer(Rae et al., 2020)は新しい圧縮記憶を追加する.
Ernie-Doc(Ding et al., 2021)は,先行するセグメントの前の層の出力にAttentionする代わりに,同じ層の再帰を採用することで,文脈的な情報の流れを強化する.
Memformer(Wu et al., 2022a)は,以前の隠れた状態を要約した表現で保存するための専用記憶モジュールを導入している.
Memformerと同様のアプローチで,MART(Lei et al., 2020)はLSTM(Hochreiter and Schmidhuber, 1997)とGRU(Cho et al., 2014)に類似した記憶更新ルールを採用する.
FeedBack Transformer(Fan et al., 2020)は,セグメントレベルを超える完全な再帰性を実装している.
既存のリカレント手法の多くは,アーキテクチャを変更する必要があり,様々な事前訓練済みモデルへの適用が複雑であるという欠点がある.
これに対して,Recurrent Memory Transformerは,共通にサポートされるインターフェースを使用するあらゆるモデル上に構築することが可能である.
いくつかのアプローチは,入力カバレッジ損失を最小限に抑えながら計算量を減らすためにSelf-Attention機構を再設計する.
Star-Transformer(Guo et al., 2019),Longformer(Beltagy et al., 2020),GMAT(Gupta and Berant, 2020),Extended Transformer Construction(ETC)(Ainslie et al., 2020),Big Bird(Zaheer et al., 2020)はAttention距離を制限し,グローバル表現などの技術を利用して長距離依存性を保持する.
Memory Transformer(Burtsev et al., 2020)は,不変のモデル入力を特別な記憶トークンで拡張することによって記憶を導入する.
これらの手法に共通する制約として,訓練と推論の両方で入力サイズに応じて記憶要件が増大するため,ハードウェアの制約により必然的に入力のスケーリングが制限されることが挙げられる.
それぞれの論文で報告されている最長のLongformer,Big Bird,Long T5(Guo et al., 2022)のモデルの最大長は33,000トークン未満である.
CoLT5(Ainslie et al., 2023)は記憶不足になる前に最大64,000トークンを処理でき,Memorizing Transformers(Wu et al., 2022b)はk近傍法によってさらに記憶を拡張する.
7章:Discussion(ディスカッション)
Transformersにおける長文入力の問題は,このアーキテクチャの普及以来,盛んに研究されてきた.
本研究では,Transformersを長文に適用しても,必ずしも大量の記憶を必要としないことを実証する.
リカレントアプローチと記憶を採用することで,2次関数的な複雑さを線形に減らすことができる.
さらに,十分に大きな入力で訓練されたモデルは,その能力を桁違いに長いテキストに外挿することができる.
本研究で検討された合成タスクは,言語モデリングを含む未知の特性を持つタスクにRMTを汎化するための最初のマイルストーンとなるものである.
今後の課題として,再帰記憶を最もよく使われるTransformerに適用し,その有効なコンテキストサイズを改善することを目指す.
References(参考文献)
- (Ainslie et al., 2020) Joshua Ainslie, Santiago Ontanon, Chris Alberti, Philip Pham, Anirudh Ravula, and Sumit Sanghai. Etc: Encoding long and structured data in transformers, 2020.
- (Ainslie et al., 2023) Joshua Ainslie, Tao Lei, Michiel de Jong, Santiago Ontañón, Siddhartha Brahma, Yury Zemlyanskiy, David Uthus, Mandy Guo, James Lee-Thorp, Yi Tay, Yun-Hsuan Sung, and Sumit Sanghai. Colt5: Faster long-range transformers with conditional computation, 2023.
- (Beltagy et al., 2020) Iz Beltagy, Matthew E Peters, and Arman Cohan. Longformer: The long-document transformer. arXiv preprint arXiv:2004.05150, 2020.
- (Bulatov et al., 2022) Aydar Bulatov, Yury Kuratov, and Mikhail Burtsev. Recurrent memory transformer. In S. Koyejo, S. Mohamed, A. Agarwal, D. Belgrave, K. Cho, and A. Oh, editors, Advances in Neural Information Processing Systems, volume 35, pages 11079–11091. Curran Associates, Inc., 2022. URL https://proceedings.neurips.cc/paper_files/paper/2022/file/47e288629a6996a17ce50b90a056a0e1-Paper-Conference.pdf.
- (Burtsev et al., 2020) Mikhail S Burtsev, Yuri Kuratov, Anton Peganov, and Grigory V Sapunov. Memory transformer. arXiv preprint arXiv:2006.11527, 2020.
- (Cho et al., 2014) Kyunghyun Cho, Bart van Merriënboer, Dzmitry Bahdanau, and Yoshua Bengio. On the properties of neural machine translation: Encoder–decoder approaches. In Proceedings of SSST-8, Eighth Workshop on Syntax, Semantics and Structure in Statistical Translation, pages 103–111, Doha, Qatar, October 2014. Association for Computational Linguistics. doi: 10.3115/v1/W14-4012. URL https://aclanthology.org/W14-4012.
- (Dai et al., 2019) Zihang Dai, Zhilin Yang, Yiming Yang, Jaime Carbonell, Quoc Le, and Ruslan Salakhutdinov. Transformer-XL: Attentive language models beyond a fixed-length context. In Proceedings of the 57th Annual Meeting of the Association for Computational Linguistics, pages 2978–2988, Florence, Italy, July 2019. Association for Computational Linguistics. doi: 10.18653/v1/P19-1285. URL https://aclanthology.org/P19-1285.
- (Devlin et al., 2019) Jacob Devlin, Ming-Wei Chang, Kenton Lee, and Kristina Toutanova. BERT: Pre-training of Deep Bidirectional Transformers for Language Understanding. In Proceedings of the 2019 Conference of the North American Chapter of the Association for Computational Linguistics: Human Language Technologies, Volume 1 (Long and Short Papers), pages 4171–4186, 2019. URL https://aclweb.org/anthology/papers/N/N19/N19-1423/.
- (Ding et al., 2021) SiYu Ding, Junyuan Shang, Shuohuan Wang, Yu Sun, Hao Tian, Hua Wu, and Haifeng Wang. ERNIE-Doc: A retrospective long-document modeling transformer. In Proceedings of the 59th Annual Meeting of the Association for Computational Linguistics and the 11th International Joint Conference on Natural Language Processing (Volume 1: Long Papers), pages 2914–2927, Online, August 2021. Association for Computational Linguistics. doi: 10.18653/v1/2021.acl-long.227. URL https://aclanthology.org/2021.acl-long.227.
- (Fan et al., 2020) Angela Fan, Thibaut Lavril, Edouard Grave, Armand Joulin, and Sainbayar Sukhbaatar. Addressing some limitations of transformers with feedback memory. arXiv preprint arXiv:2002.09402, 2020.
- (Graves et al., 2014) Alex Graves, Greg Wayne, and Ivo Danihelka. Neural turing machines. arXiv preprint arXiv:1410.5401, 2014.
- (Graves et al., 2016) Alex Graves, Greg Wayne, Malcolm Reynolds, Tim Harley, Ivo Danihelka, Agnieszka Grabska-Barwi´nska, Sergio Gómez Colmenarejo, Edward Grefenstette, Tiago Ramalho, John Agapiou, Adrià Puigdomènech Badia, Karl Moritz Hermann, Yori Zwols, Georg Ostrovski, Adam Cain, Helen King, Christopher Summerfield, Phil Blunsom, Koray Kavukcuoglu, and Demis Hassabis. Hybrid computing using a neural network with dynamic external memory. Nature, 538(7626):471–476, October 2016. ISSN 00280836. URL http: //dx.doi.org/10.1038/nature20101.
- (Grefenstette et al., 2015) Edward Grefenstette, Karl Moritz Hermann, Mustafa Suleyman, and Phil Blunsom. Learning to transduce with unbounded memory, 2015.
- (Gulcehre et al., 2016) Caglar Gulcehre, Sarath Chandar, Kyunghyun Cho, and Yoshua Bengio. Dynamic neural turing machine with soft and hard addressing schemes. arXiv preprint arXiv:1607.00036, 2016.
- (Gulcehre et al., 2017) Caglar Gulcehre, Sarath Chandar, and Yoshua Bengio. Memory augmented neural networks with wormhole connections. arXiv preprint arXiv:1701.08718, 2017.
- (Guo et al., 2022) Mandy Guo, Joshua Ainslie, David Uthus, Santiago Ontanon, Jianmo Ni, Yun-Hsuan Sung, and Yinfei Yang. LongT5: Efficient text-to-text transformer for long sequences. In Findings of the Association for Computational Linguistics: NAACL 2022, pages 724–736, Seattle, United States, July 2022. Association for Computational Linguistics. doi: 10.18653/v1/2022.findings-naacl.55. URL https://aclanthology.org/2022.findings-naacl.55.
- (Guo et al., 2019) Qipeng Guo, Xipeng Qiu, Pengfei Liu, Yunfan Shao, Xiangyang Xue, and Zheng Zhang. Star-transformer. In Proceedings of the 2019 Conference of the North American Chapter of the Association for Computational Linguistics: Human Language Technologies, Volume 1 (Long and Short Papers), pages 1315–1325, Minneapolis, Minnesota, June 2019. Association for Computational Linguistics. doi: 10.18653/v1/N19-1133. URL https://aclanthology.org/N19-1133.
- (Gupta and Berant, 2020) Ankit Gupta and Jonathan Berant. Gmat: Global memory augmentation for transformers. arXiv preprint arXiv:2006.03274, 2020.
- (Hochreiter and Schmidhuber, 1997) Sepp Hochreiter and Jürgen Schmidhuber. Long short-term memory. Neural Comput., 9(8):1735–1780, November 1997. ISSN 0899-7667. doi: 10.1162/neco.1997.9.8.1735. URL https://doi.org/10.1162/neco.1997.9.8.1735.
- (Hoffmann et al., 2022) Jordan Hoffmann, Sebastian Borgeaud, Arthur Mensch, Elena Buchatskaya, Trevor Cai, Eliza Rutherford, Diego de Las Casas, Lisa Anne Hendricks, Johannes Welbl, Aidan Clark, Thomas Hennigan, Eric Noland, Katherine Millican, George van den Driessche, Bogdan Damoc, Aurelia Guy, Simon Osindero, Karén Simonyan, Erich Elsen, Oriol Vinyals, Jack Rae, and Laurent Sifre. An empirical analysis of computeoptimal large language model training. In S. Koyejo, S. Mohamed, A. Agarwal, D. Belgrave, K. Cho, and A. Oh, editors, Advances in Neural Information Processing Systems, volume 35, pages 30016–30030. Curran Associates, Inc., 2022. URL https://proceedings.neurips.cc/paper_files/paper/2022/file/c1e2faff6f588870935f114ebe04a3e5-Paper-Conference.pdf.
- (Joulin and Mikolov, 2015) Armand Joulin and Tomas Mikolov. Inferring algorithmic patterns with stack-augmented recurrent nets, 2015.
- (Lei et al., 2020) Jie Lei, Liwei Wang, Yelong Shen, Dong Yu, Tamara L. Berg, and Mohit Bansal. Mart: Memory-augmented recurrent transformer for coherent video paragraph captioning, 2020.
- (Loshchilov and Hutter, 2019) Ilya Loshchilov and Frank Hutter. Decoupled weight decay regularization. In International Conference on Learning Representations, 2019. URL https://openreview.net/forum?id=Bkg6RiCqY7.
- (McCulloch and Pitts, 1943) Warren S McCulloch and Walter Pitts. A logical calculus of the ideas immanent in nervous activity. The bulletin of mathematical biophysics, 5(4):115–133, 1943.
- (Meng and Rumshisky, 2018) Yuanliang Meng and Anna Rumshisky. Context-aware neural model for temporal information extraction. In Proceedings of the 56th Annual Meeting of the Association for Computational Linguistics (Volume 1: Long Papers), pages 527–536, 2018.
- (OpenAI, 2023) OpenAI. Gpt-4 technical report, 2023.
- (Pang et al., 2022) Richard Yuanzhe Pang, Alicia Parrish, Nitish Joshi, Nikita Nangia, Jason Phang, Angelica Chen, Vishakh Padmakumar, Johnny Ma, Jana Thompson, He He, and Samuel Bowman. QuALITY: Question answering with long input texts, yes! In Proceedings of the 2022 Conference of the North American Chapter of the Association for Computational Linguistics: Human Language Technologies, pages 5336–5358, Seattle, United States, July 2022. Association for Computational Linguistics. doi: 10.18653/v1/2022.naacl-main.391. URL https://aclanthology.org/2022.naacl-main.391.
- (Rae et al., 2016) Jack W Rae, Jonathan J Hunt, Tim Harley, Ivo Danihelka, Andrew Senior, Greg Wayne, Alex Graves, and Timothy P Lillicrap. Scaling memory-augmented neural networks with sparse reads and writes, 2016.
- (Rae et al., 2020) Jack W. Rae, Anna Potapenko, Siddhant M. Jayakumar, Chloe Hillier, and Timothy P. Lillicrap. Compressive transformers for long-range sequence modelling. In International Conference on Learning Representations, 2020. URL https://openreview.net/forum?id=SylKikSYDH.
- (Stephen, 1956) C Stephen. Kleene. representation of events in nerve nets and finite automata. Automata studies, 1956.
- (Sukhbaatar et al., 2015) Sainbayar Sukhbaatar, Arthur Szlam, Jason Weston, and Rob Fergus. End-to-end memory networks, 2015.
- (Vaswani et al., 2017) Ashish Vaswani, Noam Shazeer, Niki Parmar, Jakob Uszkoreit, Llion Jones, Aidan N Gomez, Łukasz Kaiser, and Illia Polosukhin. Attention is All you Need. In Advances in neural information processing systems, pages 5998–6008, 2017. URL https://proceedings.neurips.cc/paper_files/paper/2017/hash/3f5ee243547dee91fbd053c1c4a845aa-Abstract.html.
- (Werbos, 1990) Paul J Werbos. Backpropagation through time: what it does and how to do it. Proceedings of the IEEE, 78(10): 1550–1560, 1990.
- (Weston et al., 2015) Jason Weston, Sumit Chopra, and Antoine Bordes. Memory networks. In Yoshua Bengio and Yann LeCun, editors, 3rd International Conference on Learning Representations, ICLR 2015, San Diego, CA, USA, May 7-9, 2015, Conference Track Proceedings, 2015. URL https://arxiv.org/abs/1410.3916.
- (Weston et al., 2016) Jason Weston, Antoine Bordes, Sumit Chopra, and Tomás Mikolov. Towards ai-complete question answering: A set of prerequisite toy tasks. In Yoshua Bengio and Yann LeCun, editors, 4th International Conference on Learning Representations, ICLR 2016, San Juan, Puerto Rico, May 2-4, 2016, Conference Track Proceedings, 2016. URL https://arxiv.org/abs/1502.05698.
- (Wolf et al., 2020) Thomas Wolf, Lysandre Debut, Victor Sanh, Julien Chaumond, Clement Delangue, Anthony Moi, Pierric Cistac, Tim Rault, Rémi Louf, Morgan Funtowicz, et al. Transformers: State-of-the-art natural language processing. In Proceedings of the 2020 conference on empirical methods in natural language processing: system demonstrations, pages 38–45, 2020.
- (Wu et al., 2022a) Qingyang Wu, Zhenzhong Lan, Kun Qian, Jing Gu, Alborz Geramifard, and Zhou Yu. Memformer: A memoryaugmented transformer for sequence modeling. In Findings of the Association for Computational Linguistics: AACL-IJCNLP 2022, pages 308–318, Online only, November 2022a. Association for Computational Linguistics. URL https://aclanthology.org/2022.findings-aacl.29.
- (Wu et al., 2022b) Yuhuai Wu, Markus Norman Rabe, DeLesley Hutchins, and Christian Szegedy. Memorizing transformers. In International Conference on Learning Representations, 2022b. URL https://openreview.net/forum?id=TrjbxzRcnf-.
- (Zaheer et al., 2020) Manzil Zaheer, Guru Guruganesh, Kumar Avinava Dubey, Joshua Ainslie, Chris Alberti, Santiago Ontanon, Philip Pham, Anirudh Ravula, Qifan Wang, Li Yang, and Amr Ahmed. Big bird: Transformers for longer sequences. In H. Larochelle, M. Ranzato, R. Hadsell, M.F. Balcan, and H. Lin, editors, Advances in Neural Information Processing Systems, volume 33, pages 17283–17297. Curran Associates, Inc., 2020. URL https://proceedings.neurips.cc/paper_files/paper/2020/file/c8512d142a2d849725f31a9a7a361ab9-Paper.pdf.
- (Zhang et al., 2022) Susan Zhang, Stephen Roller, Naman Goyal, Mikel Artetxe, Moya Chen, Shuohui Chen, Christopher Dewan, Mona Diab, Xian Li, Xi Victoria Lin, Todor Mihaylov, Myle Ott, Sam Shleifer, Kurt Shuster, Daniel Simig, Punit Singh Koura, Anjali Sridhar, Tianlu Wang, and Luke Zettlemoyer. Opt: Open pre-trained transformer language models. ArXiv, abs/2205.01068, 2022.
Scaling Transformer to 1M tokens and beyond with RMTの解説動画
Scaling Transformer to 1M tokens and beyond with RMTの解説動画です.
まとめ
Scaling Transformer to 1M tokens and beyond with RMTの日本語訳を紹介しました.
100万トークン以上をサポートしたTransformerがわかりました.
AIのプログラミング言語「C++/Python言語」を学べるおすすめのWebサイトを知りたいあなたはこちらからどうぞ.
独学が難しいあなたは,AIを学べるオンラインプログラミングスクール3社で自分に合うスクールを見つけましょう.後悔はさせません!
国内・海外のAIエンジニアのおすすめ求人サイトを知りたいあなたはこちらからどうぞ. こういった悩みにお答えします. こういった私が解説していきます. 国内・海外のAIエンジニアのおすすめ求人サイト(転職エージェント)を紹介します. AIエンジニアになるためには,主にC++/Pytho ... 続きを見る
国内・海外のAIエンジニアのおすすめ求人サイト【転職エージェント】【C++/Python言語】
国内・海外のプロンプトエンジニアのおすすめ求人サイトを知りたいあなたはこちらからどうぞ.